How to Integrate Design Thinking into Machine Learning for Innovative and Human Centric Solutions
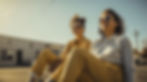
Integrating design thinking into machine learning pipelines can lead to more innovative and human centric AI solutions. This approach delivers high-performing models that align with human values by emphasising empathy and user needs. This article explores the potential benefits and challenges of combining these methodologies.
As someone from an executive background rather than a traditional AI one, I recognised the profound impact machine learning could have on businesses. This prompted me to embark on a learning journey - starting with Andrew Ng's Machine Learning course and expanding into deep learning and cloud certifications.
Equipped with these new skills, I was eager to merge business strategy and AI. But further into my journey, I encountered design thinking - an approach focused on deeply understanding user needs. This sparked my curiosity: Could combine AI and design thinking to lead to more innovative and human centred solutions?
People interact with machine learning models in various ways, and each person has different comfort levels and expectations. As a result, it's crucial that the model continuously evolves by incorporating new feedback to maintain its effectiveness and efficiency.
What is a Machine Learning Pipeline
A machine learning pipeline automates creating, training, assessing, and deploying models. These stages include Data processing, feature engineering, and model evaluation. By incorporating design thinking into this pipeline, machine learning development becomes more authentic and immersive. Addressing real-world problems while aligning with people's needs and expectations.
Unveiling the Power of Design Thinking
Design thinking focuses on deeply understanding user needs to craft desirable solutions. This human centered design approach involves empathy, prototyping, and iteration. By incorporating this creative problem solving framework into ML pipelines, we can build models that address real-world problems while prioritizing the human experience.
Let us explore how to apply design thinking to a machine learning pipeline.
Framing the Problem in the Machine Learning Pipeline
Framing the problem around user needs and perspectives is key to ML pipeline success. Asking "why?", "what if?" and "how?" questions uncovers challenges and opportunities. Problem framing is an iterative process that evolves as you gather more customer insights. This human centred approach results in solutions that address real issues. Remember that as you collect more information about the problem and your customers, you may need to revisit and adjust your questions to ensure they remain relevant and practical.
The Role of Empathy in Enhancing Machine Learning Solutions
Empathy, including interviews and observation, is vital in design thinking. Understanding users' perspectives and pain points provides insights that can inform data preprocessing and feature engineering. This human centred approach enhances ML solutions. For example, interviews with users may uncover needs around simplifying data entry that can tell how data is labelled and preprocessed.
Building Trust through Design Thinking: A Spotify Case Study
Spotify integrated design thinking when developing its machine learning music recommendation system.
By going beyond a one-size-fits-all approach and understanding users' contextual "why" behind music choices, they adjusted the algorithms to incorporate individual preferences and needs.
This human centred approach built transparency and reliability, establishing trust in the ML system. The example demonstrates how design thinking can connect technology with human experiences.
Capitalising on Domain Expertise for Effective Machine Learning Solutions
Domain expertise workshops play a crucial role in developing successful machine learning solutions. These workshops provide a co-creation space where people can deeply understand the problem area, identify critical challenges, and discover opportunities. In addition, by collaborating with domain experts, people can uncover industry nuances and specific needs.
In summary, by empathising with people through observation, interviews and domain expertise workshops, your team can create a machine learning pipeline that genuinely addresses people's needs and expectations. This human centric approach is vital for developing meaningful and impactful solutions.
Ideation in the Machine Learning Pipeline
The ideation phase is critical for generating creative ML solutions. Brainstorming sessions on algorithms, data sources, feature engineering, and model architectures spur innovation. While embracing creativity, acknowledge constraints like computing needs and model interpretability. For example, limited computing resources may require simpler models and creative data augmentation techniques. Create an open culture that facilitates sharing unconventional ideas to meet user needs.
Balancing Divergent and Convergent Thinking for Effective Ideation
Effective ideation involves divergent thinking to generate creative ideas and convergent thinking to narrow down and select the best options. For example, you could brainstorm a list of machine learning models to solve a particular problem. You could also brainstorm a list of various features to train a machine learning model. This exploration process enables the innovation of tailored solutions.
Leveraging Prototyping for Improved Machine Learning Solutions
Prototyping allows testing ML ideas early to get user feedback. For example, basic models like K-means clustering, logistic regression, and neural nets can be prototyped on sample datasets to assess performance. K-means clustering is a model that groups data points with similar characteristics. Prototyping prevents costly mistakes down the line. Multiple prototypes enable comparing approaches to select the optimal model. Overall, prototyping improves suitability and accuracy.
Testing and Iterating in the Machine Learning Pipeline
Testing and iterating are crucial to ensure the solutions meet people's needs and expectations. Design thinking provides a framework for gathering feedback from people to refine solutions.
After training a machine learning model, it's evaluated using relevant metrics. These include accuracy, precision, recall, or F1 score, validated against people's needs and goals. At this point, designers collaborate with people and stakeholders to gather feedback on the models. Testing and iterating are essential because they allow you to reduce the risk of failure by testing your designs early and often. Save time and money by making changes to your designs early.
The iterative process involves comparing the performance of different machine learning models. The selection process finally chooses the model with the best performance.
Getting a deep understanding of the solution and its customers is essential.
This understanding helps to identify the correct problems to solve and the right solutions to release. It also ensures people's needs and expectations align with the machine learning model. This understanding may lead to going back to previous stages in the design thinking process.
Navigating the Implementation of Machine Learning Solutions
The implementation stage deploys ML models into production. It involves data preparation steps like cleaning and transformation and technical deployment to cloud or on-premise systems. But most importantly, this stage centres human needs by considering user perspectives and expectations. Keeping the end-user in focus is key for real-world applications.
Incorporate diverse perspectives to address ethical concerns and increase model fairness.
Key Considerations for Implementing Human Centric Machine Learning Solutions
Model Monitoring - Continuously monitor model performance and user needs to identify issues like bias and improve.
Explainability - Increase model transparency through collaboration to build trust and acceptance.
Trust - Boost trust by explaining conclusions and emphasising empathy for users.
Ethics - Incorporate diverse perspectives to address ethical concerns and increase model fairness.
Complementing Traditional Methods - Using design thinking principles with traditional ML methods enhances development.
Responsible Oversight - ML models have limitations despite design thinking efforts. Accountable governance remains critical.
By integrating explainability, trust, and ethics, design thinking helps create responsible and transparent solutions with a positive societal impact.
Conclusion
Integrating design thinking with machine learning provides a potent, human centred approach that can complement and enhance traditional ML methods. With thoughtful coordination of human and ML capabilities, we can create solutions that address real-world issues while promoting human values. However, human oversight remains critical, as models have blindspots despite the best design thinking efforts. It bridges the gap between technology and humanity by prioritising empathy and people's experiences. The result is impactful and meaningful solutions that address real problems and improve people's lives.
Are you ready to integrate Design Thinking into your AI strategy and unlock impactful and meaningful solutions?
Visionary Leaders Choose David Kolb Consultancy. Dive into a world where business strategy meets innovative problem-solving skills. Harness the creativity needed for today's artificial intelligence in business era. Your journey to a human-centred business transformation starts with a 1:1 session.
Looking for more insightful content?
Check out these related blog posts on business innovation, design thinking, AI and technology written by David Kolb.
Or check out our weekly concise and valuable quick tips for visionary leaders and entrepreneurs.